GeneXproTools 4.0 implements the Sensitivity/Specificity/PPV/NPV
(SSPN) fitness function both with and
without parsimony pressure. The
version with parsimony
pressure puts a little pressure on the size of the evolving
solutions, allowing the discovery of more compact models.
For all classification problems, in order to be able to apply a particular fitness function,
the learning algorithms of GeneXproTools 4.0 must convert the value returned by the evolved model into “1” or “0” using the
0/1 Rounding Threshold. If the value returned by the evolved model is equal to or greater than the rounding threshold, then the record is classified as “1”, “0” otherwise.
Thus, the 0/1 Rounding Threshold is an integral part of all fitness functions used for classification and must be appropriately set in the Settings Panel -> Fitness Function Tab.
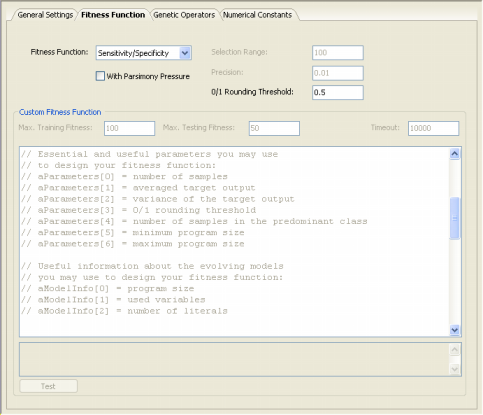
The SSPN fitness function of GeneXproTools 4.0
is, as expected, based on all the four statistical indexes:
sensitivity, specificity,
positive predictive value (PPV), and
negative predictive value (NPV).
The sensitivity/specificity/PPV/NPV SSPNi of an individual program
i is evaluated by the equation:

where SEi is the sensitivity, SPi the specificity, PPVi the positive predictive value, and
NPVi the negative predictive value of the individual program
i, and are given by the formulas:
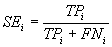
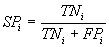


where TPi, TNi, FPi, and
FNi represent, respectively, the number of true
positives, true negatives, false positives, and false
negatives.
TPi, TNi, FPi, and
FNi are the four different possible outcomes of a single prediction for a two-class case with classes “1” (“yes”) and “0” (“no”). A
false positive is when the outcome is incorrectly classified as “yes” (or “positive”), when it is in fact “no” (or “negative”). A
false negative is when the outcome is incorrectly classified as negative when it is in fact positive.
True positives and true negatives are obviously correct classifications.
Keeping track of all these possible outcomes is such an error-prone activity, that they are usually shown in what is called a
confusion matrix. And for all classification problems, regardless of the fitness function,
GeneXproTools 4.0 shows the confusion matrix for all the evolved
models and updates it continuously during the run.
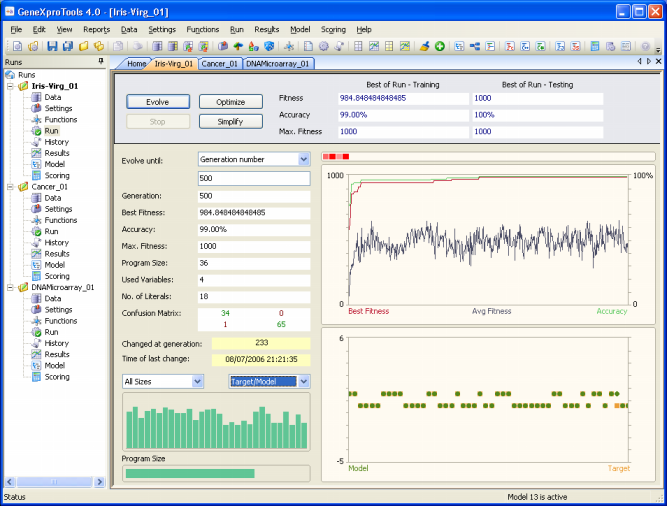
Thus, for evaluating the fitness fi of an individual program
i, the following equation is used:
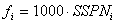
which obviously ranges from 0 to 1000, with 1000 corresponding to the ideal.
Its counterpart with parsimony pressure, uses this fitness
measure fi
as raw fitness rfi and complements
it with a parsimony term.
Thus, in this case, raw maximum fitness rfmax =
1000.
And the overall fitness fppi (that is, fitness with parsimony pressure) is evaluated by the formula: 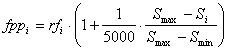
where Si is the size of the program, Smax and
Smin represent, respectively, maximum and minimum program sizes and are evaluated by the formulas:
Smax = G (h + t)
Smin = G
where G is the number of genes, and h and t are the head and tail sizes (note that, for simplicity, the linking function was not taken into account). Thus, when
rfi = rfmax and Si =
Smin (highly improbable, though, as this can only happen for very simple functions as this means that all the sub-ETs are composed of just one node),
fppi = fppmax, with fppmax evaluated by the formula:
|