In data mining, be it performed by learning algorithms or conventional statistical methods, it really pays to take a good look at your data before embarking on a complex, usually time consuming modeling
process. It's true that evolutionary algorithms are particularly well equipped to deal with noisy data, but the better the data you feed them the better the models they produce.
GeneXproTools helps you find missing and invalid (usually nominal) values in your data sets and prompts you to fix them before they are used for modeling. But the preparation of a well balanced data set should be done before loading the data into
GeneXproTools, and we recommend you to particularly take care of the following:
- Avoid using duplicated samples for they can bias the modeling process considerably.
- Choose a well balanced data set.
- Choose a reasonable number of samples for training.
An excessively large data set will slow the modeling process unnecessarily. If you have access to huge data bases it’s good practice to use the surplus samples for testing instead. A good rule of thumb consists of using about 8-10 samples for each independent variable in your training data.
Check your data sets carefully for inaccurate values. Typographical or measurement errors generally cause outliers that can be detected by graphing one variable at a time.
And you can do that easily in the GeneXproTools environment.
Data cleaning is a time-consuming and labor-intensive procedure, but one that is absolutely necessary for successful modeling. The
graphical visualization tools of
GeneXproTools 4.0 make it easy to identify outliers, which may well represent errors in the data files. After loading your data into
GeneXproTools, in the Data Panel you can visualize the distribution of values for each variable and also plot each independent variable against the dependent variable, or each attribute against the class value for classification problems.
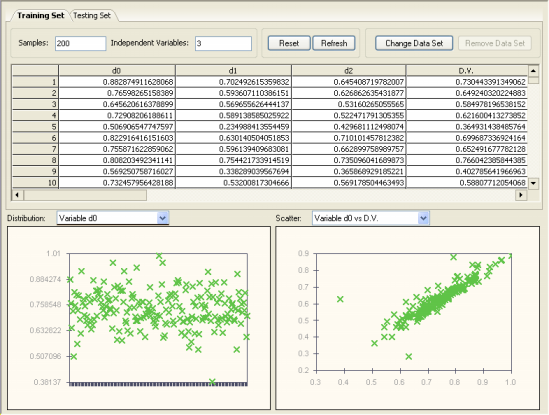
|