This indicator comes from the medical domain and consists of the probability of the diagnostic test finding no disease among those who do not have the disease or the proportion of people free of a disease who have a negative test.
More formally, the specificity SPi of an individual program
i is evaluated by the equation:
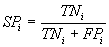
where TPi, TNi, FPi, and
FNi represent, respectively, the number of true
positives, true negatives, false positives, and false
negatives.
TPi, TNi, FPi, and
FNi are the four different possible outcomes of a single prediction for a two-class case with classes “1” (“yes”) and “0” (“no”). A
false positive is when the outcome is incorrectly classified as “yes” (or “positive”), when it is in fact “no” (or “negative”). A
false negative is when the outcome is incorrectly classified as negative when it is in fact positive.
True positives and true negatives are obviously correct classifications.
Keeping track of all these possible outcomes is such an error-prone activity, that they are usually shown in what is called a
confusion matrix. And for all classification problems, APS 3.0 shows the confusion matrix for all the evolved models.
|