Sensitivity/Specificity Fitness Function
The Sensitivity/Specificity fitness function is based on the
sensitivity and
specificity.
The sensitivity/specificity SSi of an individual program
i is evaluated by the equation:

where SEi is the sensitivity and SPi is the specificity of the individual program
i, and are given by the formulas:
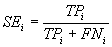
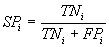
where TPi, TNi, FPi, and
FNi represent, respectively, the number of true
positives, true negatives, false positives, and false
negatives.
True positives (TP), true negatives (TN),
false positives (FP), and false negatives (FN), are the four
different possible outcomes of a single prediction for a
binomial classification task with classes “1” (“yes”) and “0” (“no”). A
false positive is when the outcome is incorrectly classified as “yes” (or “positive”),
when it is in fact “no” (or “negative”). A
false negative is when the outcome is incorrectly classified as negative when
it is in fact positive.
True positives and true negatives are obviously correct classifications.
These four types of classifications are usually shown in a two-way table called the
confusion matrix.
The Sensitivity/Specificity fitness function can be combined
with a cost matrix in order to impose specific constraints on the
solutions.
In addition, the evolvable
logistic threshold, which is intrinsic to the
logistic regression model, can be adjusted by changing the number of bins.
See Also:
Related Tutorials:
Related Videos:
|