SSPN Fitness Function
The SSPN fitness function
combines four different statistics: the
sensitivity,
specificity,
positive predictive value (PPV), and the
negative predictive value (NPV).
The SSPP measure SSPNi of an individual model
i is evaluated by the equation:

where SEi is the sensitivity, SPi the specificity, PPVi the positive predictive value, and
NPVi the negative predictive value of the individual program
i, and are given by the formulas:
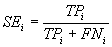
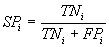


where TPi, TNi, FPi, and
FNi represent, respectively, the number of true
positives, true negatives, false positives, and false
negatives.
True positives (TP), true negatives (TN),
false positives (FP), and false negatives (FN), are the four
different possible outcomes of a single prediction for a
binomial classification task with classes “1” (“yes”) and “0” (“no”). A
false positive is when the outcome is incorrectly classified as “yes” (or “positive”),
when it is in fact “no” (or “negative”). A
false negative is when the outcome is incorrectly classified as negative when
it is in fact positive.
True positives and true negatives are obviously correct classifications.
These four types of classifications are usually shown in a two-way table called the
confusion matrix.
The SSPN fitness function can be combined
with a cost matrix in order to impose specific constraints on the
solutions.
In addition, by choosing different
rounding thresholds,
different fitness functions can be created to explore different solution spaces.
The evolvable rounding thresholds include the ROC threshold, the logistic, class proportions, large margin, average, RMS, interdecile mean,
interquartile mean, midrange, midhinge, and trimean threshold. There are also
two kinds of fixed thresholds: the rounding threshold of the active model and
user defined thresholds.
See Also:
Related Tutorials:
Related Videos:
|